I believe above all else, the role of Growth in an organization is to learn. Of course, the objective is to learn in the service of growth but I believe that the growth role is different than many traditional roles because the role demands that you navigate through a lot of ambiguity to optimize for the fit and volume of transactions between a product and it’s customers. Learning is the best antidote to ambiguity.
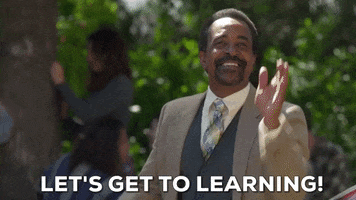
I’ve been stuck on terminology lately. The more I’ve spoken with people or read on the topic, the more interpretations of the term “growth experiment” I’ve come across. And through churning on this topic over the last couple of weeks I came up with a definition that I’m not only comfortable with, but I’m also happy with.
But before I get into that, let me share the origin of this conundrum.
Many interpretations of “Experiment”
I started in digital marketing and that isn’t the best anchoring on this topic. That space has a very narrow and very traditional definition of the term “experiment.”
The digital marketing definition of an experiment draws heavily from the scientific definition and method: you develop a hypothesis about a causal factor and a change you want to make, you group your subjects into two or more groups, you keep a control group, and you expose your experimental group(s) to some conditions, you do some statistical analysis to determine if your test conditions had an effect and you move forward accordingly.
You can run these types of experiments with digital ads, email campaigns, landing pages, and other forms of digital experiences. This is also the common conception for product designers and product managers. You can see evidence of this when you see URLs like /signup-b in a web app.
The problem with this interpretation is that it requires experimenters to be able to collect enough data to come up with a statistically valid result.
The downside of this belief are threefold:
- Data volume requirement leads you to think that they can’t run “proper experiments” if they don’t have enough data
- You have to wait for such a long time to get to statistical significance that the rate of learning renders the experimentation next to useless.
- It confines your thinking that an experiment is not an experiment if it doesn’t conform to the rigorous divide-and-expose-to-conditions structure it is not actually an experiment.
The danger of this interpretation is the fallacy that I myself have struggled with: thinking that you can’t learn if you can’t experiment.
The worst possible failure mode is to believe that the only organization capable of experimentation are those who are capturing massive volumes of data and can run a controlled test on button color with infinite precision.
Can you imagine, if only the products with over 100k users per month could run experiments? Or if businesses at the scale of Google, Facebook, and Amazon were the only businesses that experiment on anything more interesting than UI changes? This barrier would drastically stifle innovation.
A more functional definition
The crux of the definition is the weight of the structure vs intent. Where I got stuck was the definition of “experimentation” with the structure of the activity (groups and conditions) more than the intent of the activity (learning).
A more functional definition prioritizes the intent over structure. If you ask yourself, “what is an experiment if the intent of the activity is to learn?” Then the scope and structure of the activity change accordingly.
This leads me to a more functional definition:
A growth experiment is an activity that is structured in a way that the activity can provide evidence for an effect on growth and an understanding of why it had that effect.
In other words, it’s an activity that is designed to accelerate growth and has the feedback loop built in. In the face of ambiguity, a growth experiment drives a stake of certainty into the ground that suggests where you might experiment to grow into more unknown territory.
Then what becomes an experiment?
Now that the definition is focused appropriately, the scope widens significantly. Now you can fit any activity into that definition as long as you can structure the activity in such a way that it is instructive about growth.
Now the question is, “should everything be an experiment?” After thinking about it, I couldn’t come up with a reason to say “no.” Without getting into all the details, I couldn’t think of an example of an activity that was so insignificant that it shouldn’t be structured in a way that I should be able to learn from it.
If I can’t justify the effort in structuring the activity so that I can learn from it, should I take on the activity in the first place? If I won’t be able to tell if it was effective, how will I know it was effective?!
If an activity isn’t justifiable, is it justified?
Welcome to my own personal hell.
Inside the scope of an “experiment”
Run a campaign, build a feature, launch a new product, target a new market, raise prices, lower prices, start a business, kill a feature, replace a vendor, hire an employee. All these activities can be experiments but few of them fit within the scope of the A/B test definition I provided above. You’re never going to hire two employees and only intend to keep one. If you are, you should quit your job.
The common thread among all these activities is that you can develop a hypothesis about the intended effect and you can observe an initial state, observe the activity itself (especially the costs), and observe outcomes over time. You can develop an understanding of the causal relationship between these activities and your growth goals or your growth barriers.
Let’s break the structure down a bit more concretely.
How to structure a growth experiment
I think there are five parts to an experiment. And you’ll notice none of them explicitly include a wiki page, Google Analytics, or a student’s T-test. The experiment structure should be appropriate to the investment in the activity.
Determine a relationship: Observe or assume a relationship between some action and your growth goals.
“It looks like people who use the chat widget are more likely to become a customer”
Develop a hypothesis: Create a rationale for causation between affecting one side of the relationship and an expected outcome on the other.
“If we pop up the chat when we get a signal that a user is lost, they will engage with the chat, we will reduce confusion, and ultimately increase the likelihood that the user becomes a customer.”
Create and observe feedback loop: Perhaps the most important part is being intentional about the type of feedback that you collect. Your feedback must be relevant to the activity and instructive. In the examples above, traffic doesn’t matter, neither does NPS. What matters is whether the people you intend to expose to chat actually use it, if they have a good experience with the chat, and it pushes them to become a customer.
“I’m going to track the number of people that are exposed to the chat pop-up and if they engage with it. I’m going to check the transcripts of these conversations to see if they are helpful for the users. I’m also going to check to see if these users become customers.”
Now, this actually might be a case for an A/B test but it likely is not, given the number of users who are likely to be exposed to the new experience. Bear in mind that this type of longitudinal study (A for a period, then B for a period) creates some risk around bias in interpretation but the qualitative feedback from the chats can be extremely instructive in their own right.
“Post Mortem” assessment: I think post mortems are great for the learning process and for getting people on the same page, but they are a little misleading because not all experiments “die.” I look at this as just taking the opportunity to review your feedback and ask yourself (and others,) “did this action have the effect that I intended, and can I discern why?”
“Did any users engage with the chat when we intended? Did it have any effect on their experience or on their likelihood to become a customer?
Evaluation: Evaluation is different from assessment because it layers on the analysis of the outcome on top of the activity itself. This is a good time to discern some theories about growth and ask yourself, was the activity worth it? And was it likely better than other alternate activities? Should we double down or shut it down?
“We saw that the users that engaged with the chat by and large were looking for a different type of product. The additional load on our customer support team didn’t yield any favorable outcomes so we cannot justify keeping this activity running. However, we did discover some interesting insights about complementary features and how we could improve our onboarding flow”
I want to reiterate: the experiment structure should be appropriate to the investment in the activity. You never want the structure to be more cumbersome than the activity. So sometimes, the structure is just a little bit of observation and an open mindset to unexpected outcomes.
You never want to get so bogged down in observation that you slow down your rate of learning. You can think of it as an optimization between creating the most possible learning opportunities while extracting as much learning from them as possible. You’ll probably end up 80/20 on both experiment velocity and feedback structure.
Remember though, this is all about learning.